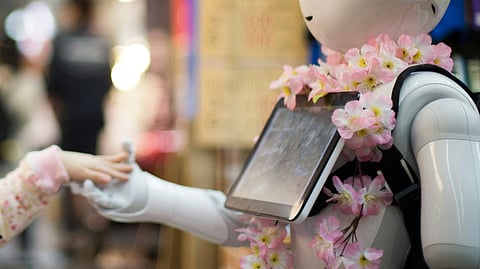
In her first year at the David Geffen School of Medicine at UCLA, Katharina “Kat” Schmolly, MD, heard an old saying: “When you hear hoofbeats, think of horses, not zebras.”
The medical maxim is a caution for physicians to prioritize likely causes rather than uncommon diagnoses. Dr. Schmolly, an undergraduate student of equine science and a former horse trainer, was on board.
But she began to reconsider during a hepatology lecture by Simon W. Beaven, MD, PhD. At his clinic in the Pfleger Liver Institute, Dr. Beaven treats patients with acute hepatic porphyria (AHP), a family of rare genetic diseases. Symptoms affect mostly women, often coinciding with the menstrual cycle, with severe, sometimes life-threatening, attacks that include abdominal pain, nausea and vomiting, limb weakness and anxiety.
“Women unfortunately get dismissed when they go to the emergency department over and over again for these complaints,” said Dr. Schmolly. “Because it looks like it's menstrual pain, but actually, it could be a true liver disease.”
She said the unfairness of it inspired her to found zebraMD (Link is external), which uses artificial intelligence to help diagnose and manage rare and genetic diseases.
A predictive algorithm combs through electronic health records to identify disease patterns and flag patients who may be at risk so physicians can further test and diagnose. The model flips the medical maxim to highlight the rare zebras over commonly known horses.
“The diagnostic delay is roughly 10 to 15 years for these diseases because physicians don't see them very often,” said Dr. Schmolly. “And while waiting for diagnosis, the disease can progress and cause irreversible damage.
"So, our goal is to diagnose patients earlier and manage their disease appropriately.”
Not so rare
By definition, a rare disease affects less than 200,000 people. Rare diseases include more than 10,000 known conditions, and cumulatively affect more than 30 million people, or 1 in 10 Americans. That’s about the same prevalence as diabetes.
A few, including multiple sclerosis, are well known. But the majority, such as bartonellosis, maple syrup urine disease and visual snow syndrome, are not.
AHP affects 1 in 100,000 people. The FDA approved givosiran in 2019 as a prophylactic treatment for recurrent attacks. But it takes an average of 15 years for diagnosis.
In an effort to speed that up, the drug’s manufacturer, Alnylam Pharmaceuticals, approached researchers at the UCSF Porphyria Center (Link is external) about developing an algorithm to identify possible patients.
“Given that it's a rare disease, we knew up front that it was going to be difficult or impossible to run this as a single center endeavor. So we looked to our peers within the UC health network, and UCLA is the largest of the centers within UC health,” said Vivek Rudrapatna, MD, PhD(Link is external), an assistant professor in the division of gastroenterology at UCSF.
When Dr. Schmolly approached Dr. Beaven about her interest in rare diseases, he connected her with Dr. Rudrapatna. He is also director of The Real-World Evidence Lab(Link is external), which applies data science techniques to electronic health records.
Together, they co-invented Project Zebra’s predictive algorithm to analyze healthcare records and identify suspected porphyria patients. Dr. Rudrapatna had trained as a clinical data scientist during his UCSF gastroenterology fellowship. Dr. Schmolly built on her pre-medical school experience with research and development at Medtronic where she worked on a diagnostic algorithm for an implantable cardiac monitoring device.
The data were de-identified patient records from UCSF and UCLA, a subset of the roughly 10 million records UC-wide. The first challenge for the algorithm: messy data. (Newswise/PL)