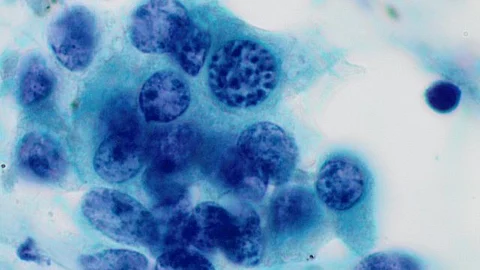
Nonfunctional pancreatic neuroendocrine tumors, while rare, are primarily treated through surgery. The presence or absence of lymph node metastasis considerably influences the selection of surgical and other treatment approaches. Particularly controversial is the necessity of surgery for tumors smaller than 2 cm as current clinical guidelines provide no clear consensus. Existing methods for preoperative diagnosis of lymph node metastasis are inadequate.
To address the aforementioned challenge, the Tsukuba team has created a predictive model by integrating radiomics features extracted from CT and MRI images using artificial intelligence deep-learning techniques. This model has demonstrated an 89% success rate in predicting lymph node metastasis, a rate that rises to 91% when the model is validated with data from an external hospital. Furthermore, the performance of the model remains consistent, irrespective of the tumor size being larger or smaller than 2 cm.
In conclusion, the model can help predict lymph node metastasis. Moreover, the model equips surgeons with a crucial tool for selecting the most appropriate surgical procedures and treatment strategies, potentially transforming patient outcomes in the challenging medical field.
###
This work was supported by JSPS KAKENHI Grant Number JP22K20814; the Rare Tumor Research Special Project of the National Natural Science Foundation of China (82141104) and Clinical Research Special Project of Shanghai Municipal Health Commission (202340123).
Original Paper
Title of original paper:
Development and validation of CT-based radiomics deep learning signatures to predict lymph node metastasis in non-functional pancreatic neuroendocrine tumors: a multicohort study. (KB/newswise)